Software tools help researchers find genetic mutations that can cause cancer
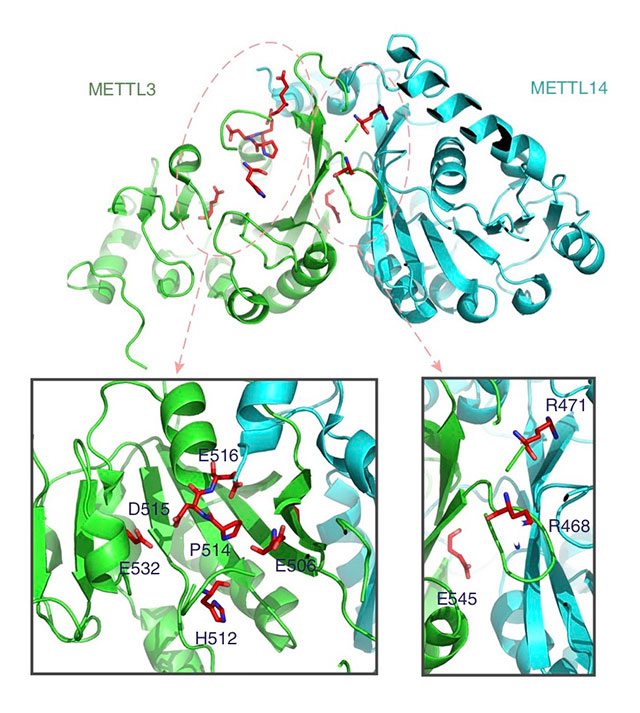
Identifying which gene mutations are most likely to propel cancer forward can help doctors treat cancer patients more effectively and help researchers better understand the biology of cancer. But finding these “driver” genes isn’t easy. Any cell can and will acquire gene mutations, but only a fraction of those aberrations have the potential to survive, proliferate and create tumors.
Driver genes tend to have higher numbers of mutations than non-driver genes. Researchers have been able to find them by sequencing the DNA of cancer tissue from many different patients and then counting the number of mutations on each gene. This method works well with some common cancers, but isn’t as effective with other malignancies because there often isn’t a large enough sample size to find a pattern in the data. It also misses a large number of potential genes that drive the formation of tumors in only a small fraction of cancer patients.
Now Xin He, PhD, assistant professor of human genetics at the University of Chicago, Matthew Stephens, professor of human genetics and statistics, and their colleagues have developed a computational software program that can tease out driver genes from non-driver genes much more effectively than previous methods. Their program, called driverMAPS (Model-based Analysis of Positive Selection), does more than just count the number of mutations on genes. It also considers the functional importance of the mutation, or how much it affects the gene’s ability to do its job.
Looking at patterns, not just counting
Driver gene mutations tend to be more deleterious — that is they may render a gene non-functional — than non-driver gene mutations. By analyzing functional importance all the way down to the base-pair level, the program is able to sift through different levels of mutational changes and give more weight to those that cause bigger functional modifications.
In addition, the program considers the spatial patterns of mutations on genes. When looking at the mutation data of multiple patients, the mutations could be spread out randomly across the same gene or they could occur around the same spot. Driver genes tend to have mutations that group together in the same area and non-driver genes tend to have mutations that are more randomly located.
If you know what genes drive your particular cancer, you can use a drug that specifically targets those genes.
“By combining this functional information and spatial clustering, our method is much more sensitive todetecting driver genes than many other models,” He said. “Our idea is to look at the patterns of the mutations, not just count the number of mutations.”
When He’s team tested their software they found, for example, a mutation that occurred in four different patients on the exact same spot on a particular gene. Programs that simply count mutations on genes might not have considered the gene as a driver gene because four mutations in hundreds of patients is not very many. But driverMAPS recognized that four mutations in the exact same spot on a particular gene was extremely unlikely to happen just by chance.
“To borrow a comment from my colleague, that’s like multiple lightning strikes hitting exactly the same spot,” He said.
Testing its METTL
The researchers tested driverMAPS through simulations and by applying it to data from The Cancer Genome Atlas, a project by the National Cancer Institute to catalog genetic mutations that are responsible for cancer. They analyzed 734,754 mutations from 20 tumor types and identified 159 new potential driver genes. From there, they chose to experimentally validate one of the genes, METTL3, which driverMAPS had identified as a driver gene for bladder cancer. Because METTL3 has a low mutation rate, other programs had not picked it out as a driver gene.
METTL3 is involved in RNA modification, or changes to the chemical composition of RNA molecules after synthesis has occurred. The driverMAPS program uncovered several mutations on METTL3 that could be involved in disrupting this modification process.
To further understand how these mutations might work to propel cancer, He and his co-authors performed several in-vitro experiments. In one, they found that the mutations on METTL3 reduced the methyltransferase activity of the gene. In another, they “knocked down” METTL3 and found that this increased the proliferation of bladder cancer cells and resulted in tumor growth.
“Our work helps establish the link between the really important biological process of RNA modification and cancer,” He said.
In the future, the researchers would like to apply driverMAPS to different settings, such as finding driver genes in pediatric cancer patients or searching for driver mutations in the non-coding genome. He said discovering more driver genes can help researchers gain new insight into how cancer cells earn an advantage over other cells in terms of proliferation and survival. In addition, it can also help physicians better personalize cancer treatment since different cancer patients develop different sets of mutations that lead to their malignancies.
“There’s a lot of interest and hope in tailoring treatment to your mutation profile,” he said. “If you know what genes drive your particular cancer, you can use a drug that specifically targets those genes.”
The driverMAPS research was published in Nature Communications. Additional authors on the study include Siming Zhao, Jun Liu, Pranav Nanga, Yuwen Liu, Nicholas Knoblauch, and Chuan He from the University of Chicago; and A. Ercument Cicek from Bilkent University, Turkey.
Citation: “Detailed modeling of positive selection improves detection of cancer driver genes,” Nature Communications. July 30, 2019. DOI: 10.1038/s41467-019-11284-9.